Research topics
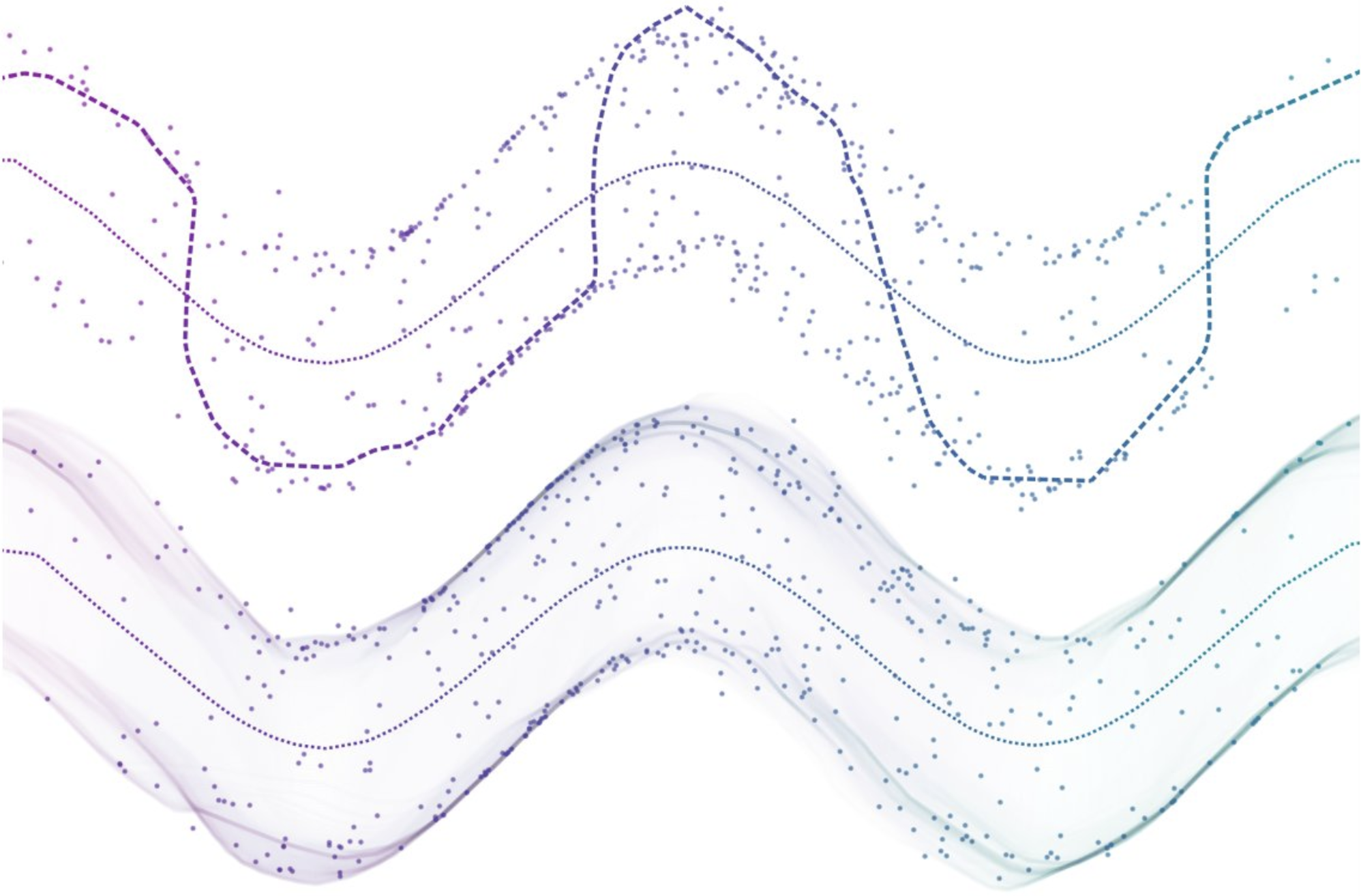
Adversarial Variational Auto-Encoder: Plumerault et al. (ICPR2020) introduce a new framework that combines VAE and GAN in a novel and complementary way to produce an auto-encoding model that combines the statistical properties of VAEs while generating realistic images of GAN-quality. Illustration of a toy example with two-dimensional data and a one-dimensional latent space. Points: data, dotted line: manifold of reconstructions from VAE, dashed line/density: manifold of reconstruction with AVAE. Color encodes the position in the one-dimensional latent space. Top: with a deterministic generator; Bottom: with a probabilistic generator. ( Collaboration with CEA-LIST, H. Le Borgne)
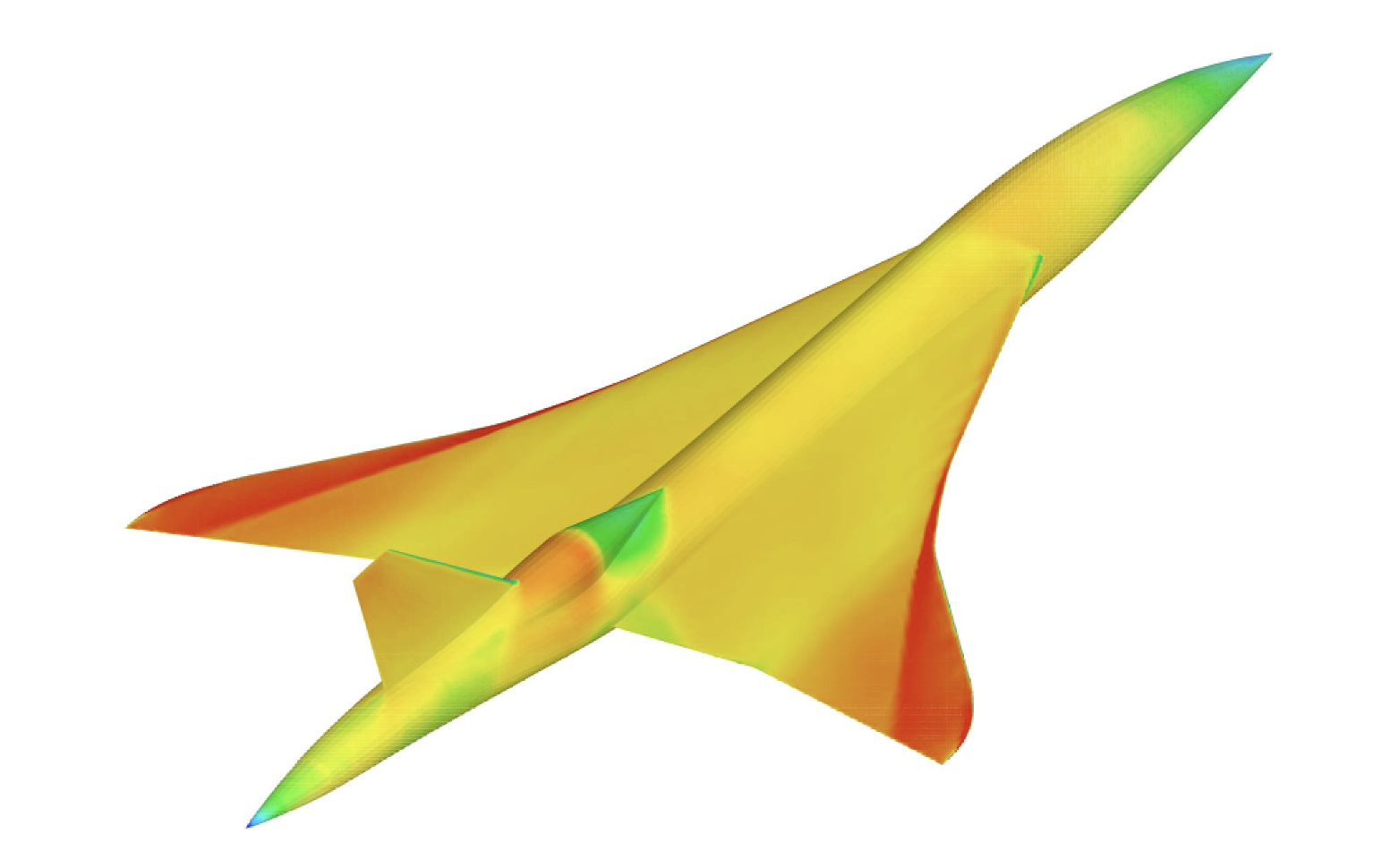
Parallel computational fluid dynamics : In fluid dynamics, turbulence is a fluid motion charaterized by chaotic changes in pressure and flow velocity. The vorticity of a flow field is a vector field that gives a microscopic measure of local rotation of the fluid. This image illustrates the numerical simulation of the vorticity on an airplane geometry occurring during the optimization process of the shape of the wing in order to reduce turbulence around the airplane.
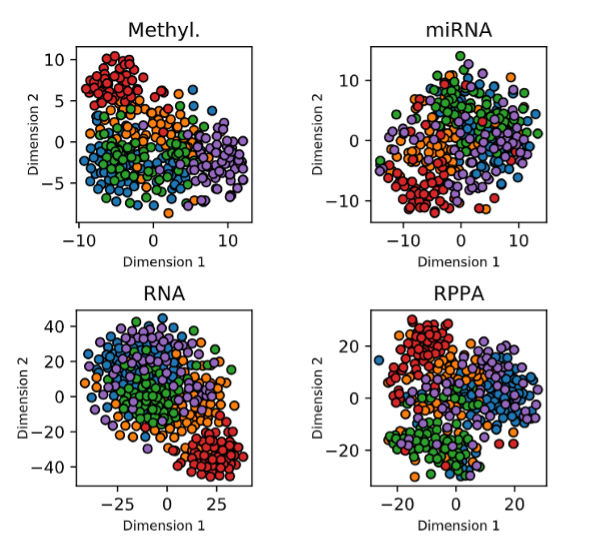
Clustering of Breast Cancer Patients from Multi-Omics Data (Trascriptomics - RNA and microRNA, Epigenetics, Proteomics) with deep-learning. We show above the tsne projection of the clustering result for each omic source, highlighting a good integration of the defferent datatypes and their usefulness in the identification of subtypes. The representation from which the clusters were computed were obtained by stacking hierarchical autoencoders with layer-wise reconstructions loss. To learn more: Viaud G, Mayilyahanan P, PH Cournede (2021). representation Learning for the Clustering of Multi-Omics Data. IEEE/ACM transactions on computational biology and bioinformatics.
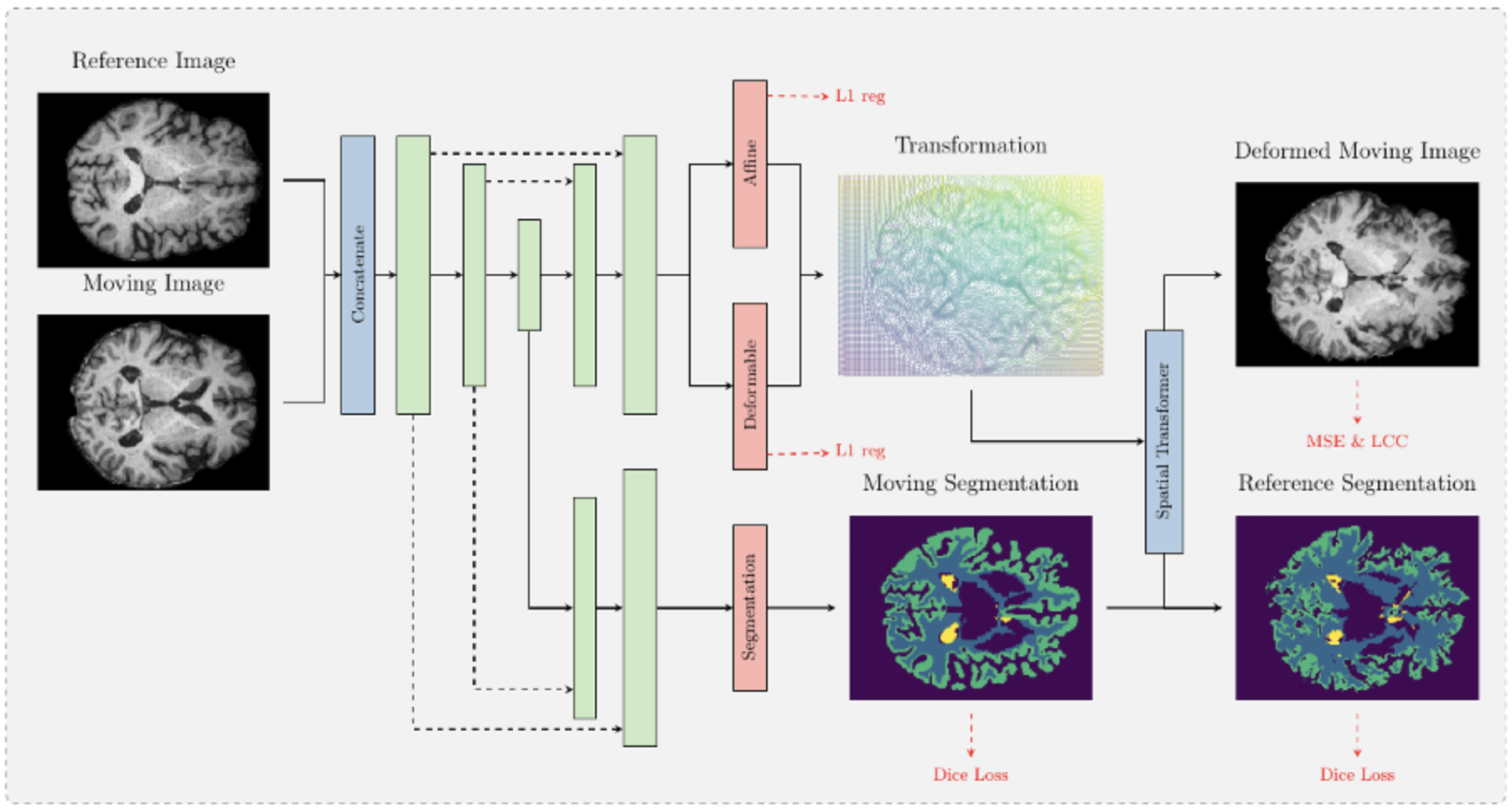
Medical Imaging (UResnet) : We propose a novel 3D deep neural network called U-ReSNet, a joint framework that can accurately register and segment medical volumes. The propsed network learns to automatically generate linear and elastic deformation models. In parallel, a coupled architectre is integrated, seeking to provide segmentation maps for anatomies or tissue patterns using an additional decoder part trained with the dice coefficient metric. Our experiments with brain MR images indicate the potentials of our method which is composed from a convolutional architecture that is extremely simple and light in terms of parameters.
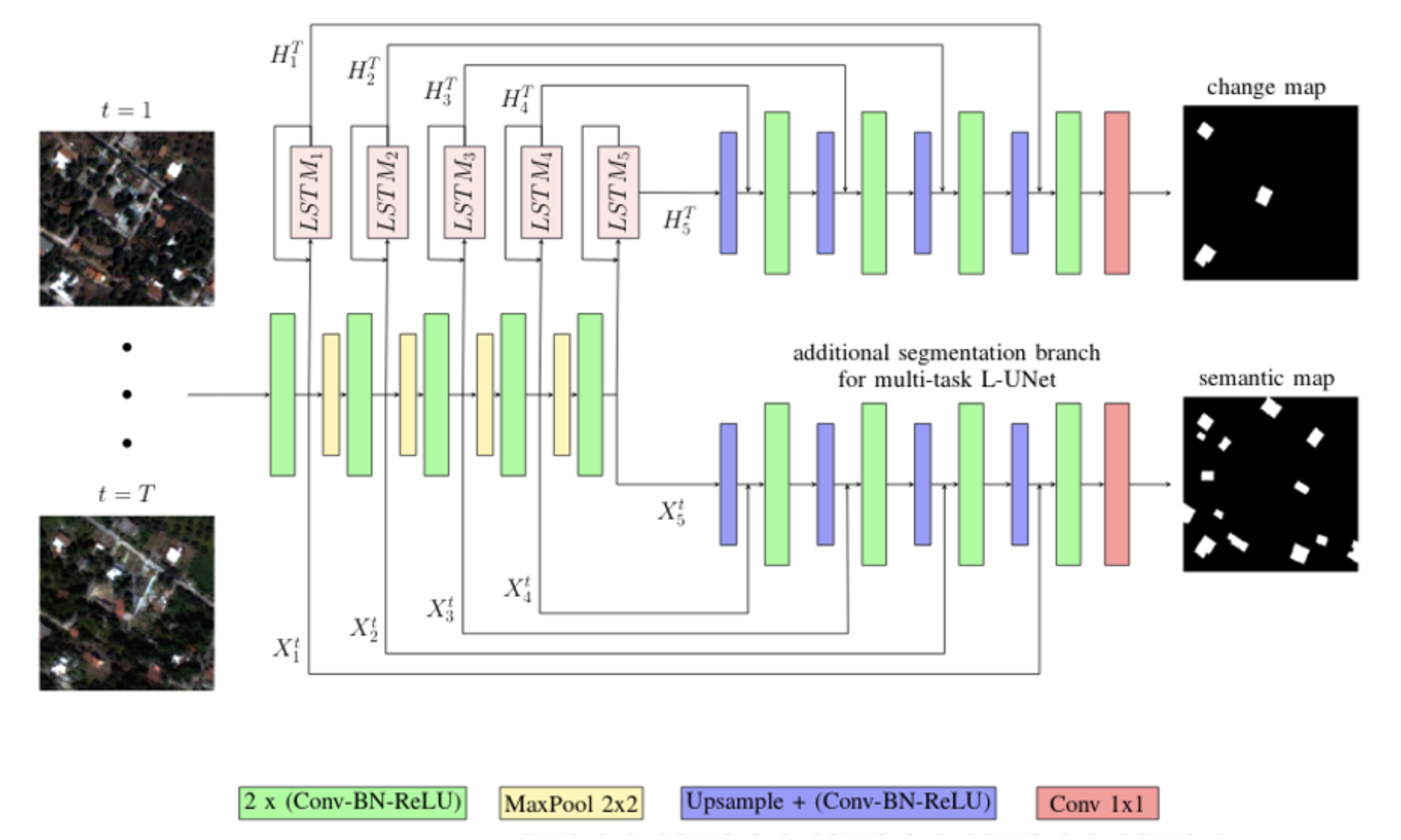
A Deep Multi-Task Learning Framework Coupling Semantic Segmentation and Fully-Convolutional LSTM Network for Urban Change Detection : A deep multi-task learning framework is presented in order to tackle the problem of urban change detection in high and very high resolution satellite images. The proposed scheme is able to couple semantic segmentation with fully convolutional long short-term memory(LSTM) networks. Regarding the fully convolutional LSTM structure, it has been designed by replacing the gating mechanisms with convolutional layers. The goal here is to combine spectral and spacial information, while taking advantage of the temporal relationship among the feature matrices avoiding the computationally expensive task of multiplying high dimensional feature vectors. The fully convolutional LSTM blocks are placed on top of each encoding level of a UNet-like deep architecture, capturing in this way temporal information for all the different resolutions levels. In addition, an extra decoding branch is integrated for the semantic segmentation of the available categories, providing the network with fruitful supplementary feature attributes during the training procedure. An ensemble of losses combined in a circular way is also employed for the optimization process. The developed methodology is evaluated on three datasets with different spatial and temporal resolution. PhD of Maria Papadomanolaki under the supervision of Maria Vakalopoulou and Konstantinos Karantzalos (NTU Athens), https://hal.inria.fr/hal-03140492
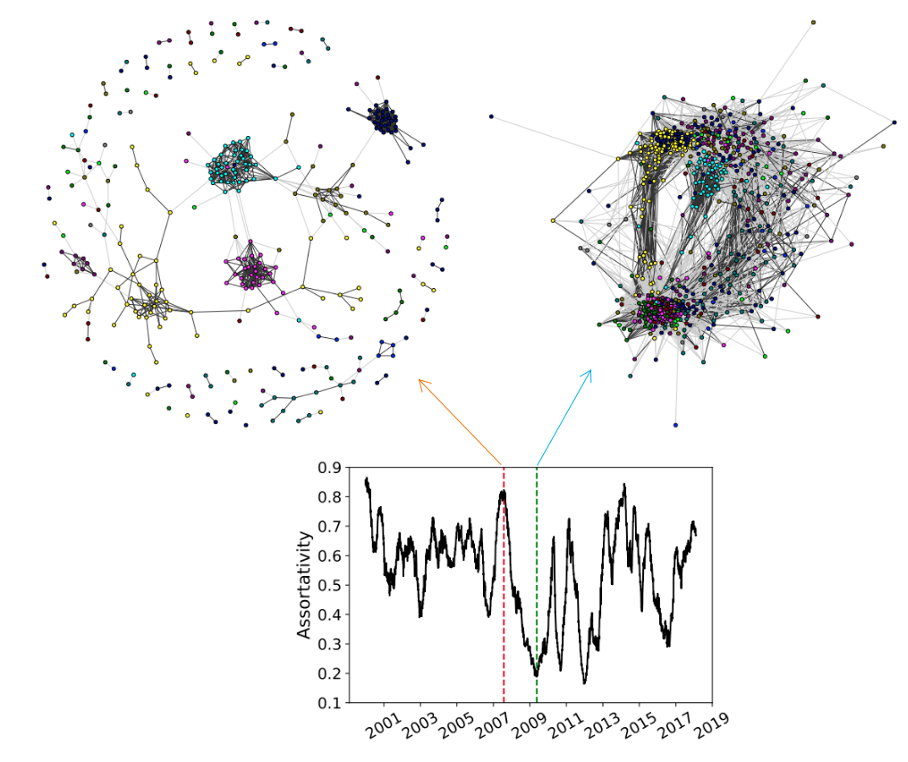
Dynamical evolution of the topological structure of equity correlation matrices : The color code of the nodes represents the economic sector partition. The propensity to establish links within the same economis sector can be measured with the assortativity coefficient, which highlights the fragility of the observed partition. In particular, during the financial crisis, the system collapses into a bipolar structure, with approximately two giant clusters of stocks highly interconnected. (https://arxiv.org/pdf/2001.11214.pdf)
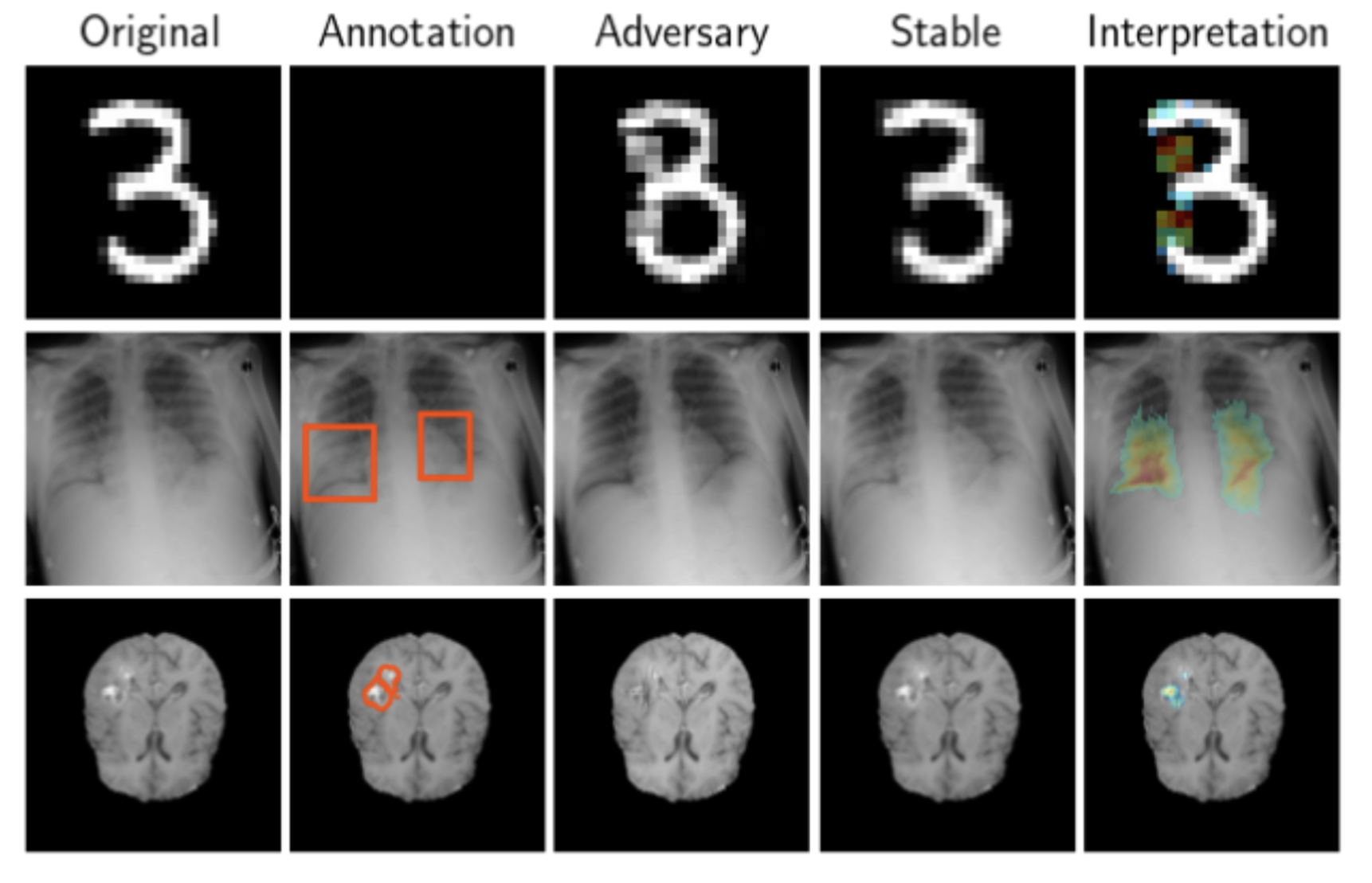
Leveraging Conditional Generative Models in a General Explanation Framework of Classifier Decisions: From left to right for the MNIST digit classification, Chest X-Rays pneumonia detection and Brain MRI tumor localization: the original image; the ground annotations pointing out the relevant regions for humans; the adversarial image (for the classifier to explain); the stable image; our resulting explanation map of the classifier's decision. PhD. of Martin Charachon, a collaboration with Incepto-Medical (Dr. Roberto Ardon).